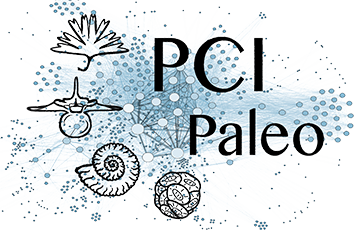
Advances in understanding how stratigraphic structure impacts inferences of phenotypic evolution
Identification of the mode of evolution in incomplete carbonate successions
Abstract
Recommendation: posted 01 July 2024, validated 03 July 2024
Hopkins, M. (2024) Advances in understanding how stratigraphic structure impacts inferences of phenotypic evolution. Peer Community in Paleontology, 100289. 10.24072/pci.paleo.100289
Recommendation
A fundamental question in evolutionary biology and paleobiology is how quickly populations and/or species evolve and under what circumstances. Because the fossil record affords us the most direct view of how species lineages have changed in the past, considerable effort has gone into developing methodological approaches for assessing rates of evolution as well as what has been termed “mode of evolution” which generally describes pattern of evolution, for example whether the morphological change captured in fossil time series are best characterized as static, punctuated, or trending (e.g., Sheets and Mitchell, 2001; Hunt, 2006, 2008; Voje et al., 2018). The rock record from which these samples are taken, however, is incomplete, due to spatially and temporally heterogenous sediment deposition and erosion. The resulting structure of the stratigraphic record may confound the direct application of evolutionary models to fossil time series, most of which come from single localities. This pressing issue is tackled in a new study entitled “Identification of the mode of evolution in incomplete carbonate successions” (Hohmann et al., 2024).
The “carbonate successions” part of the title is important. Previous similar work (Hannisdal, 2006) used models for siliciclastic depositional systems to simulate the rock record. Here, the authors simulate sediment deposition across a carbonate platform, a system that has been treated as fundamentally different from siliciclastic settings, from both the point of view of geology (see Wagoner et al., 1990; Schlager, 2005) and ecology (Hopkins et al., 2014). The authors do find that stratigraphic structure impacts the identification of mode of evolution but not necessarily in the way one might expect; specifically, it is less important how much time is represented compared to the size and distribution of gaps, regardless of where you are sampling along the platform. This result provides an important guiding principle for selecting fossil time series for future investigations.
Another very useful result of this study is the impact of time series length, which in this case should be understood as the density of sampling over a particular time interval. Counterintuitively, the probability of selecting the data-generating model as the best model decreases with increased length. The authors propose several explanations for this, all of which should inspire further work. There are also many other variables that could be explored in the simulations of the carbonate models as well as the fossil time series. For example, the authors chose to minimize within-sample variation in order to avoid conflating variability with evolutionary trends. But greater variance also potentially impacts model selection results and underlies questions about how variation relates to evolvability and the potential for directional change.
Lastly, readers of Hohmann et al. (2024) are encouraged to also peruse the reviews and author replies associated with the PCI Paleo peer review process. The discussion contained in these documents touch on several important topics, including model performance and model selection, the nature of nested model systems, and the potential of the forwarding modeling approach.
References
Hannisdal, B. (2006). Phenotypic evolution in the fossil record: Numerical experiments. The Journal of Geology, 114(2), 133–153. https://doi.org/10.1086/499569
Hohmann, N., Koelewijn, J. R., Burgess, P., and Jarochowska, E. (2024). Identification of the mode of evolution in incomplete carbonate successions. bioRxiv, 572098, ver. 4 peer-reviewed by PCI Paleo. https://doi.org/10.1101/2023.12.18.572098
Hopkins, M. J., Simpson, C., and Kiessling, W. (2014). Differential niche dynamics among major marine invertebrate clades. Ecology Letters, 17(3), 314–323. https://doi.org/10.1111/ele.12232
Hunt, G. (2006). Fitting and comparing models of phyletic evolution: Random walks and beyond. Paleobiology, 32(4), 578–601. https://doi.org/10.1666/05070.1
Hunt, G. (2008). Gradual or pulsed evolution: When should punctuational explanations be preferred? Paleobiology, 34(3), 360–377. https://doi.org/10.1666/07073.1
Schlager, W. (Ed.). (2005). Carbonate Sedimentology and Sequence Stratigraphy. SEPM Concepts in Sedimentology and Paleontology No. 8. https://doi.org/10.2110/csp.05.08
Sheets, H. D., and Mitchell, C. E. (2001). Why the null matters: Statistical tests, random walks and evolution. Genetica, 112, 105–125. https://doi.org/10.1023/A:1013308409951
Voje, K. L., Starrfelt, J., and Liow, L. H. (2018). Model adequacy and microevolutionary explanations for stasis in the fossil record. The American Naturalist, 191(4), 509–523. https://doi.org/10.1086/696265
Wagoner, J. C. V., Mitchum, R. M., Campion, K. M., and Rahmanian, V. D. (1990). Siliciclastic Sequence Stratigraphy in Well Logs, Cores, and Outcrops: Concepts for High-Resolution Correlation of Time and Facies. AAPG Methods in Exploration Series No. 7. https://doi.org/10.1306/Mth7510
The recommender in charge of the evaluation of the article and the reviewers declared that they have no conflict of interest (as defined in the code of conduct of PCI) with the authors or with the content of the article. The authors declared that they comply with the PCI rule of having no financial conflicts of interest in relation to the content of the article.
Funded by the European Union (ERC, MindTheGap, StG project no 101041077)
Evaluation round #2
DOI or URL of the preprint: https://www.biorxiv.org/content/10.1101/2023.12.18.572098v2
Version of the preprint: 2
Author's Reply, 30 May 2024
Decision by Melanie Hopkins
, posted 21 May 2024, validated 22 May 2024
Dear Niklas and co-authors,
Thank you for submitting the revised manuscript. Your response resolved many reviewer questions, but I decided to solicit one more review to comment specifically on the outstanding issue of model performance. Note that the reviewer was very positive about the study overall, not least of which its novelty, but also made some important clarifying statements about the modeling framework that might impact your thinking about the results and/or inspire some additional analyses. I invite you to consider these and look forward to seeing the revised manuscript.
Cheers,
Melanie
Reviewed by Gene Hunt
, 11 May 2024
This manuscript uses forward sedimentological modeling to generate synthetic stratigraphic sections, simulates trait evolution in the stratigraphic and time domains, and then fits evolutionary models to assess the degree to which stratigraphic architecture affects evolutionary interpretation. Surprisingly, they find that the geological effects are minor, but that a common way to analyze models of trait evolution seems to perform poorly, regardless of the geological filter.
There is a lot to admire about this manuscript. Explorations of how stratigraphic structure can affect interpretation of trait evolution in time-series has been almost completely ignored since Bjarte Hannisdal’s excellent paper almost 20 years ago. I don’t have the expertise to evaluate the sedimentological model, but it is well explained, and the exploration of a carbonate system is unique in the literature of evolutionary time-series, as far as I know. The study is well designed, using both idealized and empirical sea level curves, and sampling across different parts of the carbonate platform. Figures are very nice, and it is written clearly and with grace.
The one problem is that the surprisingly bad performance of the evolutionary models is based on a misunderstanding, and, as a result, a lot of the results and discussion will need to be reconsidered. The general issue is that one needs to evaluate not just model support (AICc and Akaike weights), but also model parameters to understand an analysis. In this study, specifically the use of the OU model creates confusion because this model can take on parameter values that cause it to converge to the other three models. The OU model has 4 parameters: the ancestral trait value (anc), the optimal trait value (theta), the strength of attraction to the optimum (alpha), and the stochastic component (vstep).
- When alpha is very strong, the traits are drawn very quickly to the optimum. In the limit (alpha -> Inf), you get a white noise process around theta, with a stationary variance of vstep/(2*alpha), equivalent to how stasis is modeled.
- When alpha is very weak, the optimum has little effect. As alpha -> 0, you get a random walk / Brownian motion.
- When alpha is weak and the optimum is very far from the starting trait value, you get a nearly linear trend from anc to theta.
So, the initially confusing results of evolutionary model fitting can be understood: nearly all model support is received by either the generating model or the OU model with parameter values that cause it to mimic the generating model. For example, when stasis is the generating model, theta and anc will be very similar and alpha values will be quite strong (often easier to judge from the half-life, log(2)/alpha), and furthermore, vstep/(2*alpha) will be nearly exactly equal to the stasis variance. Note that considering parameter values removes all difficulties of interpretation: the user would find that all the model support goes to two different ways to parameterize white noise, which is the correct, generating model.
The Discussion alludes to this property of the OU model (line 687ff), but doesn’t make the connection to interpreting the results in this light. The need to consider parameter values was one of the major points of Grabowski et al. (2023) in their correct criticism of Cooper et al.’s (2016) paper about OU models.
In terms of how to handle this, the paper can be revised to account for this interpretation, but I’d argue it would be better served by simply omitting the OU model, for two reasons. First, this model is not easily to justify biologically. Yes, the OU model can be used to model a population converging to a new adaptive peak. But this dynamic is rapid, and is expected to last a few generations to, at most, a few thousand generations. On the ~2 Myr scale of this study, there really isn’t any expectation that this dynamic should be captured. Second: URW, Trend/drift, and stasis are useful because they capture three qualitatively different evolutionary patterns: meandering, directional, and fluctuating, respectively. I don’t see any benefit to adding a 4th model that just has the effect of mimicking the best-supported of the other models, essentially splitting the support for the correct dynamic over two nearly equivalent models.
Another contributing factor here is in how Akaike weights work with nested models. If one model is nested within another (e.g., Brownian motion within Brownian motion with drift; the other three models are also nested or nearly nested within OU), it is impossible for the simpler model to decisively beat the more complex one according to Akaike weight. The log-likelihood of the more complex model cannot be lower than that of the simpler model when they are nested. Therefore, the only way for the simpler model to be better is via the parsimony term in AIC. For models that differ by 1 parameter, this leads to a delta AIC of 2, and maximum Akaike weights of 0.73 for the simple model, even when the simple model is correct (see Hunt 2006, p. 596). This is for AIC; with AICc, the exact weight will be initially higher for the simple model and then converge to the AIC value with increasing n. This means that the 0.9 used as a threshold for Akaike weight is inappropriate: it is mathematically impossible for the simpler of nested models to reach this threshold for AIC (and for AICc except when the parsimony penalty is high at low n). This, by the way, also explains the puzzling behavior in Figure 10 in which performance seems to gets worse with increasing n: more complex models will face decreasing parsimony penalties as n increases, which explains the asymptotic increase in support for OU in these plots.
I will say that I am puzzled that the OU model so consistently beats Stasis even with the two extra parameters in the OU model. It doesn’t really matter much here because the dynamics will be basically equivalent (as discussed above), but this is something I am curious about.
Below I have added some minor comments, in manuscript order. Despite the problem I have identified above, I want to emphasize how much I like this study. With suitable revision, I think it will be an important contribution to the literature.
Minor comments, in manuscript order
- Line 34: here, and elsewhere in the manuscript, pulsed change is referred to as punctuated equilibrium. I don’t think this is quite accurate: the punc eq model has pulsed change but it occurs at lineage splitting. A pulsed change within an unbranched lineage is more evidence against than for punc eq because it involves large changes without speciation. (Gould would sometimes try to cloud this issue.) I’d recommend using terms like pulsed or punctuated change, and not punctuated equilibrium, for unbranched lineages.
- Line 88, before the Fossilized Birth Death model and related approaches, there was a phase in which fossil data was used a lot (sometimes naively) to get constraints for node dating approaches.
- Line 110ff: The presentation of completeness that I am familiar with (e.g., Shanan Peters’ work) emphasizes that completeness will depend on the temporal scale of resolution. A section may be mostly complete when considered in 1 Myr bins, but will be much less so if the bins are 10 Kyr.
- Line 169: here and at a few other places, it seems to imply that previous approaches in paleo have required samples to be equally spaced in time. The model fitting approaches used here and in Hunt (2006) cited here have always allowed for arbitrary spacing of samples.
- Line 305: I don’t think it needs to be done in this paper, but, as an FYI, it is not difficult to generate realizations of the OU model with unequal sampling. The sim.OU function in paleoTS does it one way, and there is another approach in which a whole time-series is a single draw from a multivariate normal distribution using the vector of means and covariance matrix from Hansen & Martins (1996).
- Line 322: not quite right as written, as the standard deviation would be sigma *sqrt(t), not sigma. The simulation code is correct, though.
- I would not say scenario 3 is “weakly directional”. Both it and scenario 4 are strongly directional, really more so than just about any empirical sequence. This can be seen from the figures – both look almost like straight lines -- and the results are basically the same throughout for both. Calculations from Hunt (2012, Table 1) indicate that directionality accounts for 98% and 99.5% of the evolutionary change in scenario 3 and 4, respectively. I’d recommend just keeping scenario 3 as representing trends and dropping the unrealistic scenario 4.
- Line 348ff. I see the need for the distinction, but it seems odd to call them both time-series. Perhaps instead they can be stratophenetic series and time-series? The former phrase has been used occasionally in this literature.
- Line 516, about stratigraphic completeness not being the driver of outcomes. This is an interesting and important point.
- Line 640ff: this section should be reconsidered based on my comments above. The discussion about Levy flights is interesting, as I agree that kind of dynamic would probably be favored when there are unrecognized hiatuses in a section. That model isn’t implemented in paleoTS, but (within-lineage) punctuations are.
- Line 826: this references Hannisdal (2006). The only other example of a similar study I know of appears in one of the chapters of the Patzkowsky and Holland book. I think that should be cited somewhere in here as well. (It is possible that chapter refers to another paper that I am not remembering at the moment, too.)
- Line 829: you would probably see more artefactual support for stasis if the generating parameters didn’t have such high rates. With lower rates, sampling noise would be a larger component, and since sampling noise looks like stasis, you should get more spurious cases of stasis. Analyzing more shorter sequences might have a similar effect.
- I love the forward modeling approach to investigate what happens under known conditions. I am curious if you think these sedimentation models might ever be used for the inverse problem, so as to generate more realistic age models for empirical fossil time-series?
Signed,
Gene Hunt
References
Hansen, T. F., and E. P. Martins. 1996. Translating between microevolutionary process and macroevolutionary patterns: the correlation structure of interspecific data. Evolution 50(4):1404-1417.
Hunt, G. 2012. Measuring rates of phenotypic evolution and the inseparability of tempo and mode. Paleobiology 38(3):351-373.
Patzkowsky, M. E., and S. M. Holland. 2012. Stratigraphic Paleobiology: Understanding the Distribution of Fossil Taxa in Space and Time. University of Chicago Press, Chicago.
Download the reviewEvaluation round #1
DOI or URL of the preprint: https://doi.org/10.1101/2023.12.18.572098
Version of the preprint: 1
Author's Reply, 24 Mar 2024
Decision by Melanie Hopkins
, posted 29 Feb 2024, validated 01 Mar 2024
Dear Niklas and co-authors,
This project tackles an important issue in the study of phenotypic evolution using fossil data: that the structure of the stratigraphic record confounds the direct application of evolutionary models to these data. This is underexplored (and somewhat ignored) but is exactly the type of work paleobiologists need to be doing. Please find below three reviews from researchers who are very familiar with the evolutionary modeling side of things and/or the sedimentary modeling side of things. I think these comments indicate both the merit of the work but also the need for some revisions if this is going to be as impactful as it could be.
To this end, I was also particularly surprised by the performance of the model selection without stratigraphic biases. One reviewer’s comments about the need for the continuous-time expansion may be relevant here. But I also wonder if the results that you are getting are due at least in part to using model implementations for testing (those from the paleoTS package) which are different from the implementations used to simulate the data (those original to this study). To quickly assess this, I ran some simulations using the functions available in the paleoTS package. I found some interesting patterns and they are not completely different from what you found. For example, short time series (N=5) simulated under the stasis model in paleoTS are hard to distinguish from unbiased random walks (and this is expected given the smaller number of parameters in the unbiased random walk model). Similarly there is increasing support for OU as time series length increases. However, stasis never “loses” to the same degree as shown in your Figure 10. In fact, stasis is the best model in the majority of cases across all time series lengths*. It is also worth noting that the results are sensitive to the amount of variance (stasis is more strongly supported for time series simulated with smaller variance). I have included the R script and figure here in a PDF (which is the file type I can upload; I'm happy to send the actual files to you directly).
*Although rarely with an AICc > 0.9. One reviewer commented on the stringency (and interpretation) of relying on AICc values that high, which I think is worth considering. Another reviewer wondered about using AICc instead of AIC. Based on my knowledge of the paleoTS package and your R scripts (utils.R), you must have used AICc -- so this should be clarified in the text.
I look forward to seeing the revised manuscript!
Download recommender's annotationsReviewed by Bjarte Hannisdal, 21 Feb 2024
Thank you for the opportunity to comment on this very interesting manuscript by Hohmann et al. Their study revisits a long-standing problem in paleobiology: to what extent can we disentangle the true signals of evolutionary processes from the convoluted signals of processes involved in creating the strata in which the fossils occur. More specifically, the authors address the problem of characterizing morphological trait evolution ('tempo and mode') from carbonate successions. Their approach is to filter stochastic models of trait evolution through simulated stratigraphy and subject the resulting records to an established method for analyzing trait evolution in paleobiology. The authors find that for their chosen simulation scenarios, and using a support threshold criterion for model identification, this method fails to identify the underlying model of trait evolution from stratigraphic records. Remarkably, they report that even in the absence of any stratigraphic distortions, the true mode cannot be detected. Hence, they starkly conclude that a mainstream analytical tool for characterizing trait evolution in paleobiology is unable to correctly identify the mode of evolution even when provided with true time series data without any stratigraphic biases.
The authors should be commended for confronting the difficult issues that arise when considering paleobiological data in their stratigraphic context, especially at high spatiotemporal resolution. I think it is fair to say that these issues have been treated a bit too casually in some of the paleobiological literature. When discussing stratigraphic biases, they rightly point out that the main concern is not overall stratigraphic incompleteness per se (in the sense of a total ratio of deposition to non-deposition), but rather the irregularity of the gap distribution. Although the questions have been asked before, and addressed in similar ways, the authors make several novel contributions by targeting carbonate systems, and by taking a slightly different approach to implementing the canonical 'random walk' models of trait evolution.
Ultimately, however, I find some key parts of this manuscript a bit confusing, and it is unclear to me how they justify some of the choices they make. I am also not sure if they interpret their results in a way that is justified. As a consequence, I find myself questioning the extent to which their findings support their strong conclusion.
Simulation of trait evolution (p. 14-16)
When simulating the three canonical 'modes' of trait evolution (stasis, unbiased random walk, and biased random walk), the authors state that they cannot use discrete random walk models with equidistant time steps because the time elapsed between the sampling positions in the simulated stratigraphy can vary by orders of magnitude. Therefore, they use continuous models (Brownian motion/drift, in their terminology; aka Wiener process) that can be sampled at arbitrary points in time.
That's fine, but it seems like an unnecessary complication. Presumably, the relevant temporal resolution is the time step of the CarboCAT simulations, represented as discrete time bins in the chronostratigraphic (Wheeler) diagrams in Figure 3. Discrete random-walk models would model the net change in a trait from one time step to the next, which would seem to do the job just fine. At any spatial grid cell, the trait evolution would only be sampled stratigraphically in those time bins in which CarboCAT shows deposition of relevant facies.
The authors then state that the reason why they excluded Ornstein-Uhlenbeck models from their simulations is because they couldn't generate samples unequally spaced in time. But if an OU process is a modified (mean-reverting) continuous Wiener process, then why can it not be sampled at arbitrary points in time? Without further explanation, I don't understand why this would be a problem.
On page 15, the authors describe how they use the age-depth relationship from the CarboCAT simulations to map between the stratigraphic domain and the time domain, and that this transformation is done using a software package previously published by the first author. The use of some formal notation in this paragraph (e.g. a morphism) hints at a theoretical framework underpinning this software. I do not doubt that the authors have good reasons for applying this framework and the R package for age-depth mapping. However, those reasons are not made sufficiently clear in this manuscript. Why is this software needed? Intuitively, the CarboCAT simulation output (Figs. 2 & 3) provides the age-depth mapping, as well as the relevant temporal and stratigraphic (sampling) resolution. What does this additional software bring to the table, and does it have any role in the other modeling choices made in this study (e.g. the need for continuous models or for excluding OU)?
The authors simulate somewhat extreme versions of the different modes of trait evolution, representing stasis as a pure white noise process, and directional evolution as near-monotonic trends. One could argue that these end-member patterns are appropriate in the sense that they would bias against the findings: the more clear-cut the patterns, the easier it should be for the analytical methods to correctly identify them. On the other hand, there is a certain risk of venturing outside the realm of the plausible. For example, we don't need recourse to modeling to see that if trait evolution is a monotonic, linear trend, then the magnitude of stratigraphic gaps will be proportional to the jumps in trait value. By restricting within-sample trait variance and setting a fixed sample size, the authors minimize the effects of sampling error on the trait mean, which would render directional patterns more random, and random patterns more static. This effect would shift the distribution of observed evolutionary modes towards stasis, which is consistent with empirical results from paleobiological data, but excluded from this study.
Time domain analyses
To investigate the effect of stratigraphic architecture, the authors perform the model indentification procedure on time series of trait evolution "without any losses or stratigraphic distortions". In their description of this time domain analysis (p. 18, lines 366-374) it is not clear how this time domain sampling is performed, and I initially thought the sampled points were evenly distributed in time. However, the fact that they do separate time domain sensitivity analyses on the two stratigraphic scenarios, and given the description in Figure 1, I assume that the time domain analyses are performed on the points sampled in the stratigraphic domain, but that the time elapsed between samples is now part of the input to the mode identification analysis. The authors need to explain this more clearly. It would help the reader if the authors could plot examples of the position of the sampled points on the time series in some of the model realizations (e.g. Fig. 7).
Identification of the mode of trait evolution
The authors analyze the simulated fossil time series of trait evolution using Gene Hunt's paleoTS package, which computes AIC values for the three canonical modes considered by the authors, as well as an OU process. They calculate the AIC weights, which represent a measure of relative support for the different models. The authors then define a threshold value for model identification: only if the AIC weight of a single best model is > 0.9 do they consider a model to be identified. The authors attribute this threshold approach to Portet (2020), but I seem to remember that this was discussed in the book by Burnham & Anderson (2002). I may misremember, but my understanding was that if the AIC weight is >0.9, then one is justified in identifying a single best model, and otherwise one should present and discuss the relative support for multiple models. The authors instead seem to argue that if there is no single best model, then there are no supported models! I am no expert on AIC, but I'm not sure if their interpretation of "no model identified" is justified even if there is no "single best model identified".
The paleoTS analysis results shown in Figures 8 and 9 suggest that in terms of the AIC weights, the results for the stratigraphic domain and the time domain are qualitatively very similar. If the authors' interpretation of the 0.9 threshold is correct, then no models are supported in any of their analyses, and they are not justified in making further inferences. If, on the other hand, the 0.9 criterion only applies to selecting a single best model, then the authors would be justified in interpreting the relative support for different models. If the interpretation of relative model support is valid, the interpretation of their results would change substantially:
Arguably, the most striking finding is that the results for the stratigraphic domain analyses and the time domain analyses are so similar, which could mean two things: (1) The time domain data are also stratigraphically distorted to some extent because the temporal sampling is so highly irregular, which implies that their analysis is not well designed to test for the effect of stratigraphic biases per se. (2) The paleoTS analysis is actually very robust to the simulated stratigraphic distortions, which would be in sharp contrast to the authors' conclusions.
Moreover, except for the stasis simulations, the paleoTS analyses tend to show highest relative support for the correct model, particularly for the Brownian drift (GRW) models. For both the weak and strong Brownian drift, the stratigraphic domain results show greater relative support for the correct model than the time domain analysis. This is not surprising, because the very strong trends generated in these models will be condensed stratigraphically into even more extreme trends, favoring even more strongly biased random walks. As expected, the results for the Brownian motion (URW) models are less clear cut, and the counterintuitive effect of time series length may be interpretable, as discussed below.
For the stasis simulations, the paleoTS analysis clearly favors the wrong model (OU) across all the scenarios shown in this manuscript, which is obviously problematic and worth pursuing. I don't have any experience with or insight into this, but the authors may want to consult the literature on phylogenetic comparative methods that discuss issues surrounding bias towards OU models in AIC model selection (https://doi.org/10.1111/2041-210X.12285).
The sensitivity of the time domain analysis to time series length (Fig. 10) is counterintuitive, and the authors understandably struggle to make sense of it. How could increasing the number of observations in the time series reduce the relative support for the correct model? I wonder if this might be an expression of the kind of stratigraphic distortion the authors are seeking to investigate. In some of the simulations reported in Hannisdal (2006), I found that the greatest stratigraphic distortions could occur in the thickest, most densely sampled distal sections. These sections were not characterized by unusually large gaps per se, but rather by unusually high completeness of the deposits between the gaps, yielding dense temporal sampling of short time windows. The more densely these short windows were sampled, the greater the distortion, in some cases. This might be relevant for understanding the counterintuitive effect of denser sampling reported in this manuscript, if the denser sampling is constrained within short windows of deposition.
Additional comments
The aim and approach of this manuscript is quite similar to my ancient study (H06), allowing me the indulgence to reflect on the pros and cons of H06, which was part of my dissertation. On the one hand, Hohmann et al's approach is more sophisticated than H06 in terms of running multiple model realizations and scenarios, and replacing the old hypothesis tests with likelihood-based model selection. On the other hand, H06 attempted to use a biologically motivated model of trait evolution, taking into account population size, ecological preference, and preservation.
In terms of research design, however, these kinds of stochastic forward model experiments have their limitations. The space of possible models is too vast be explored exhaustively, but if done thoughtfully they can help build an intuition and a greater understanding of the problem and how to address it. It was pointed out to me back then that although understanding how stratigraphy can distort trait evolution is important, the real question is what to do about it. Already then, the field was shifting from classical null hypothesis testing towards parameter estimation and model selection. Hence, the H06 paper was merely preparing the ground for proposing a new, inverse modeling approach, part of which was published in Hannisdal (2007, Paleobiology), which I believe holds more important messages than H06. I get a sense that the authors of this manuscript also aim to set the stage for a new approach, and I look forward to seeing what they come up with.
Finally, I think the authors should engage a bit more with the literature on this long-standing problem. The introduction would greatly benefit from a more representative view of the history of this research topic. For what it's worth, I found a link to my 2006 dissertation, which includes a short introduction chapter with an overview of the literature up to that point, in the hope that the authors might find it useful. In addition to the two published papers H06 and H07, the thesis also includes an unpublished chapter on model selection:
Reviewed by anonymous reviewer 1, 13 Feb 2024
The manuscript investigates the effects of stratigraphic biases on our ability to detect the true evolutionary mode in the fossil record. The authors point out that most of the work focusing on interpreting fossil time series uses age-depth models based on simplified and highly unrealistic assumptions regarding the regularity of the stratigraphic record. This is problematic, as stratigraphic biases might affect how we interpret phenotypic change in the rock record. The manuscript is therefore addressing an important issue and has the potential to be a significant contribution to the field. However, for reasons detailed below, I am not entirely convinced by, nor sure how to interpret, the results of the manuscript.
Main comment:
It seems a bit worrying that the correct (simulated) evolutionary model is not recovered under excellent sample conditions in the absence of stratigraphic biases. A simulation study like this is, to some extent, equivalent to an experiment in the sense that you want to have a ‘control’ so that you can reliably measure the effect of the variable(s) you later start to tweak. It therefore appears imperative to recover the true evolutionary model in the simulated data in instances when the factors that you want to test the effects of later on (e.g. stratigraphic bias, etc.) are not allowed to compromise the signal in the data. The fact that it is harder to detect the true evolutionary mode with longer time series is also worrying. Analyzing more data that is generated under the true model should make detection easier. I am not convinced by the suggested reasons the authors provide to explain their lack of ability to detect the true model in absence of any stratigraphic biases in the data, and I sense (but might be wrong) the authors are not entirely convinced by their own arguments either. I thus advocate that the authors investigate why they fail to recover the true model in their data that lack stratigraphic biases, as the rest of the results in this work are difficult to interpret without a fully functioning ‘control’. If this can be fixed, or at least explained, this manuscript is likely to be a significant contribution.
Minor comments:
Figure 1: This figure is important as it describes the study design. I would have appreciated a more detailed figure caption to make it easier to understand the different steps in the study.
Line 338: Why include a sample variance? Including this will introduce noise into the data, and this is not one of the aspects under investigation.
Line 351 – 354: The rationale for including the OU model as one of the candidate models when investigating relative model fit is unclear. Wouldn't it make more sense to assess how a model of punctuated evolution performs? Such a model is available in the paleoTS package. Hiatuses of a specific duration appear to produce a punctuation-like pattern in the stratigraphic domain when the underlying model is either an unbiased or biased random walk. This test would connect the work more closely to the ongoing debate regarding the validity of the punctuated equilibrium hypothesis.
Using a criterion of 0.9 for AIC weights is quite stringent, particularly since the number of data points in each time series is relatively small, and the various models can generate largely similar trait dynamics (e.g., stasis and OU can produce very similar trait evolution). With small sample sizes, it is common to use the AICc, the bias-corrected version of the Akaike Information Criterions. AICc approaches AIC asymptotically as the sample size increases. Is there a specific reason why you have favored AIC over AICc?
FYI: I am not an expert on computer simulations of sedimentary strata, so I am not in a position to critically assess whether the simulations using the CarboCAT Lite model of carbonate platform formation are sensible.
Reviewed by Katharine Loughney
, 24 Jan 2024
This manuscript uses forward computer models to assess the fidelity of evolutionary signals preserved in stratigraphic successions forming on carbonate platforms. The motivation for the manuscript is the tendency of evolutionary (paleo)biologists to interpret the evolutionary modes of lineages at face value, rather than considering how the incompleteness of rock successions affects the preservation of evolutionary patterns. The authors examine trait lineages evolving under several evolutionary modes in two model scenarios using a simulated sea-level (SL) curve and the Pleistocene SL curve. An important finding is that the correct mode of evolution is not recovered from the modelled scenarios, and there is low statistical support for model recovery in general.
Carbonate platforms are important sources of the fossil record but have, to my knowledge, received less attention than passive margins from workers using models to analyze the preservation of stratigraphic and fossil records. I think this manuscript is compelling and well designed, and I have a few comments and suggestions. My expertise is not in evolutionary models, so my comments pertain mostly to the stratigraphic implications of the models.
General comments
Overall, the manuscript is concise and reads well, although the authors should proofread the manuscript thoroughly to check for minor grammatical issues such as subject–verb agreement and to check the formatting of citations and references.
The authors begin the manuscript with a discussion of stratigraphic bias on the fossil record. I suggest finding an alternative phrase to refer to the control of stratigraphy on the fossil record, as “bias” has negative connotations that may serve to justify the perceived shortcomings of paleontological investigations. Revealing the structure of the record (Holland, 2017) is the key to convincing neontologists to pay attention to stratigraphy. This is an important goal of studies like this, and this manuscript will be a stronger contribution toward this goal if the authors choose an alternative phrasing.
Model design: I take it that an assumption of the model is that all lineages are assumed to have an equal chance of being sampled across facies (or environments, as they are represented in the model). The authors should clarify this point in the Methods, since facies preference is another factor contributing to the “incompleteness” of the fossil record. Perhaps a follow-up study would be to examine the fidelity of evolutionary models reconstructed for lineages with distinct facies preferences in carbonate platforms.
The model constructs lineage patterns based on sampling one synthetic column at a time. I am curious whether the reconstruction of the evolutionary modes improves from tracking lineages from composite columns, similar to how graphical correlation integrates stratigraphic or biostratigraphic data from multiple locations.
The majority of the Results and Discussion focuses on general trends from scenarios A and B, and the figures almost exclusively show output from scenario A. I think it is great that the authors used the actual SL record in scenario B, but it barely comes up in the text. It is then odd when output from scenario B appears in Figure 7. I suppose the authors feel it is a moot point because the detection of evolutionary modes was not ultimately affected by SL or stratigraphic completeness, but I would like to see more discussion of the different (or similar) implications of both scenarios. I also think there is a missed opportunity to not only emphasize the relevance of the model findings to the real-world record, but also to say something about reconstructions of trait evolution from the real record.
Because the SL curves in each scenario impart different frequencies and durations of hiatuses, I think it is worth adding more emphasis of the importance of this to the real-world record. When the importance of hiatus frequency and duration is discussed in the Results (section beginning on line 485), the differences between scenarios are hinted at but not explicitly stated. If the real-world SL curve imparts many short hiatuses (and a more continuous age-depth model), then the potential to measure real modes of trait evolution is perhaps not as bad as we tend to fear because the record is “incomplete.” This point is eventually made later (lines 711–713). I think it is worth pointing this out to the reader earlier and briefly expanding on the implication that, although the real-world SL record is highly variable, it may result in a stratigraphic record that has a fairly good ability to preserve evolutionary change in the fossil record.
Tables and figures
The figures are appropriate and support the text, although I suggest a few minor modifications to some of the figures. Additionally, some of the figure captions need more explanation of the information conveyed in the figures.
In Figure 2, it would be helpful to have a color key for the different facies depicted in the simulated shelves. Differentiating among facies is not a focus of this manuscript, however, they are clearly shown in this figure and in Figure 3. In the caption, please clarify what are “the graphs,” or refer to other figures by number.
In Figure 3, please include a color key to the facies, as suggested for Figure 2. In the caption, I suggest either stating the explicit distance from shore or adding a line to the Wheeler diagrams to show where the graphs were extracted, rather than referencing the “middle” of the grid. Please clarify: the extracted “graphs” are 2C and 2F?
Figure 7 illustrates the point that stratigraphic completeness in and of itself has little bearing on the preservation of trait evolution patterns. It is unclear why a column from 2 km in scenario A is compared to a column from 6 km scenario B. Scenario B is so little discussed in the Results and Discussion, and there is little explanation for the rationale of comparing two different platform locations across the two scenarios. I think the point is that completeness doesn’t vary too much, but it is hard to take that away from comparing two completely different column locations and scenarios. Please explain this choice in the caption and text or consider modifying the figure to show outputs from more readily comparable example columns.
Figures 8 and 9, 10: Captions need explanation of the abbreviated tested modes in the legends.
I suggest adding a table to report the results of the AIC analyses for the different simulations and models. The pertinent results are reported in the text, but a table would be helpful for presenting all the AIC values.
Referred references
Holland, S.M., 2017, Presidential address: Structure, not bias: Journal of Paleontology, v. 91, p. 1315-17.
Holland, S.M., 2022, The structure of the nonmarine fossil record: Predictions from a coupled stratigraphic–paleoecological model of a coastal basin: Paleobiology, p. 1-25, doi: 10.1017/pab.2022.5
Specific comments
Line 19: The clause, “computer simulations of geological processes . . .” needs an object for “allow.”
Line 29 and elsewhere: What is meant by “adequate model”? The authors mention “adequate models” several times in the text before getting close to what they mean on pages 32 and 36. Is an adequate model highly supported statistically? Does an adequate model faithfully simulate the mode of evolution? Please define this term in the Introduction and add a brief explanation in the Abstract.
Line 71: This phrasing makes it sound like sedimentology and stratigraphy are the only disciplines that are jargon laden. It is a fair point that sed/strat—and many disciplines—are often written for narrow audiences that may make it difficult for outsiders to glean relevant information. I think the issue is more that evolutionary biologists are typically interested in ages of fossils that can be used to calibrate trees, not their stratigraphic contexts. They don’t know that the stratigraphy informs the age estimate and they do not know to look for it.
Line 94: Fossil-bearing stratigraphic successions.
Line 203: Were run
Line 289: Awkward wording
Line 295: “. . . trait value that describes”
Lines 658–660: Yes, it is important to examine multiple columns along dip for interpreting the patterns. The authors may want to acknowledge here that this is a bit of an oversimplification that may not directly apply to carbonate platforms. Given that the geometry of carbonate platforms causes hiatuses to form along dip, similar age-depth models should be recovered from neighboring columns. In practice, it may be difficult to compare platform locations to the distal slope locations, and I expect the facies and fossil assemblages would be very different. During lowstands, sediment may accumulate on the slope, but it will be redeposited material that may not preserve fossils well, making it difficult to sample the traits of interest.
Line 691: “. . . jumps will coincide with gaps . . .”
Line 716: “First” (avoid adding -ly in writting)
Line 723: “Second”
Line 778: I’m confused by the use of “ground truthing” here or by the wording of this sentence. Are the authors suggesting that the models offer ground truth? Ground truth can only be gotten in the field.
Lines 783–784: see also strataR in Holland (2022)
Download the review