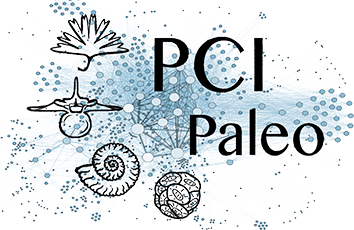
Performance of machine-learning approaches in identifying ammonoid species based on conch properties
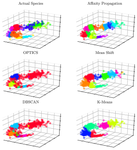
Ammonoid taxonomy with supervised and unsupervised machine learning algorithms
Abstract
Recommendation: posted 13 September 2021, validated 01 October 2021
De Baets, K. (2021) Performance of machine-learning approaches in identifying ammonoid species based on conch properties. Peer Community in Paleontology, 100010. 10.24072/pci.paleo.100010
Recommendation
There are less and less experts on taxonomy of particular groups particularly among early career paleontologists and (paleo)biologists – this also includes ammonoid cephalopods. Techniques cannot replace this taxonomic expertise (Engel et al. 2021) but machine learning approaches can make taxonomy more efficient, reproducible as well as passing it over more sustainable. Initially ammonoid taxonomy was a black box with small differences sometimes sufficient to erect different species as well as really idiosyncratic groupings of superficially similar specimens (see De Baets et al. 2015 for a review). In the meantime, scientists have embraced more quantitative assessments of conch shape and morphology more generally (see Klug et al. 2015 for a more recent review). The approaches still rely on important but time-intensive collection work and seeing through daisy chains of more or less accessible papers and monographs without really knowing how these approaches perform (other than expert opinion). In addition, younger scientists are usually trained by more experienced scientists, but this practice is becoming more and more difficult which makes it difficult to resolve the taxonomic gap. This relates to the fact that less and less experienced researchers with this kind of expertise get employed as well as graduate students or postdocs choosing different research or job avenues after their initial training effectively leading to a leaky pipeline and taxonomic impediment.
Robust taxonomy and stratigraphy is the basis for all other studies we do as paleontologists/paleobiologists so Foxon (2021) represents the first step to use supervised and unsupervised machine-learning approaches and test their efficiency on ammonoid conch properties. This pilot study demonstrates that machine learning approaches can be reasonably accurate (60-70%) in identifying ammonoid species (Foxon, 2021) – at least similar to that in other mollusk taxa (e.g., Klinkenbuß et al. 2020) - and might also be interesting to assist in cases where more traditional methods are not feasible. Novel approaches might even allow to further approve the accuracy as has been demonstrated for other research objects like pollen (Romero et al. 2020). Further applying of machine learning approaches on larger datasets and additional morphological features (e.g., suture line) are now necessary in order to test and improve the robustness of these approaches for ammonoids as well as test their performance more broadly within paleontology.
References
De Baets K, Bert D, Hoffmann R, Monnet C, Yacobucci M, and Klug C (2015). Ammonoid intraspecific variability. In: Ammonoid Paleobiology: From anatomy to ecology. Ed. by Klug C, Korn D, De Baets K, Kruta I, and Mapes R. Vol. 43. Topics in Geobiology. Dordrecht: Springer, pp. 359–426.
Engel MS, Ceríaco LMP, Daniel GM, Dellapé PM, Löbl I, Marinov M, Reis RE, Young MT, Dubois A, Agarwal I, Lehmann A. P, Alvarado M, Alvarez N, Andreone F, Araujo-Vieira K, Ascher JS, Baêta D, Baldo D, Bandeira SA, Barden P, Barrasso DA, Bendifallah L, Bockmann FA, Böhme W, Borkent A, Brandão CRF, Busack SD, Bybee SM, Channing A, Chatzimanolis S, Christenhusz MJM, Crisci JV, D’elía G, Da Costa LM, Davis SR, De Lucena CAS, Deuve T, Fernandes Elizalde S, Faivovich J, Farooq H, Ferguson AW, Gippoliti S, Gonçalves FMP, Gonzalez VH, Greenbaum E, Hinojosa-Díaz IA, Ineich I, Jiang J, Kahono S, Kury AB, Lucinda PHF, Lynch JD, Malécot V, Marques MP, Marris JWM, Mckellar RC, Mendes LF, Nihei SS, Nishikawa K, Ohler A, Orrico VGD, Ota H, Paiva J, Parrinha D, Pauwels OSG, Pereyra MO, Pestana LB, Pinheiro PDP, Prendini L, Prokop J, Rasmussen C, Rödel MO, Rodrigues MT, Rodríguez SM, Salatnaya H, Sampaio Í, Sánchez-García A, Shebl MA, Santos BS, Solórzano-Kraemer MM, Sousa ACA, Stoev P, Teta P, Trape JF, Dos Santos CVD, Vasudevan K, Vink CJ, Vogel G, Wagner P, Wappler T, Ware JL, Wedmann S, and Zacharie CK (2021). The taxonomic impediment: a shortage of taxonomists, not the lack of technical approaches. Zoological Journal of the Linnean Society 193, 381–387. doi: 10. 1093/zoolinnean/zlab072
Foxon F (2021). Ammonoid taxonomy with supervised and unsupervised machine learning algorithms. PaleorXiv ewkx9, ver. 3, peer-reviewed by PCI Paleo. doi: 10.31233/osf.io/ewkx9
Klinkenbuß D, Metz O, Reichert J, Hauffe T, Neubauer TA, Wesselingh FP, and Wilke T (2020). Performance of 3D morphological methods in the machine learning assisted classification of closely related fossil bivalve species of the genus Dreissena. Malacologia 63, 95. doi: 10.4002/040.063.0109
Klug C, Korn D, Landman NH, Tanabe K, De Baets K, and Naglik C (2015). Ammonoid conchs. In: Ammonoid Paleobiology: From anatomy to ecology. Ed. by Klug C, Korn D, De Baets K, Kruta I, and Mapes RH. Vol. 43. Dordrecht: Springer, pp. 3–24.
Romero IC, Kong S, Fowlkes CC, Jaramillo C, Urban MA, Oboh-Ikuenobe F, D’Apolito C, and Punyasena SW (2020). Improving the taxonomy of fossil pollen using convolutional neural networks and superresolution microscopy. Proceedings of the National Academy of Sciences 117, 28496–28505. doi: 10.1073/pnas.2007324117
The recommender in charge of the evaluation of the article and the reviewers declared that they have no conflict of interest (as defined in the code of conduct of PCI) with the authors or with the content of the article. The authors declared that they comply with the PCI rule of having no financial conflicts of interest in relation to the content of the article.
Reviewed by Jérémie Bardin, 08 Sep 2021
The author improved the manuscript in a satisfying way:
- an additional method has been included
- discussion has been extended to propose future developments and orientations for paleontologists
- previous minor comments have been considered.
I still think that more specimens, species and morphological traits are needed to quantify the effectiveness of these methods. This being said, such developments of quantitative taxonomy and systematics are really needed in our field, so I recommend this paper which is a very stimulating step.
Hereafter, few very minor points:
Table 1. Maybe property is not the best word. Why not just “parameter” as in the legend or “variable”?
p.2
“the” should precede “PBDB” when used as a name. Several occurrences throughout the text.
P.5
"becase" -> "because"
p.9
Future studies should seek to replicate these findings which (-> With?) richer data sources in both sample size and species count (->s).
Evaluation round #1
DOI or URL of the preprint: https://doi.org/10.31233/osf.io/ewkx9
Author's Reply, 05 Jul 2021
Decision by Kenneth De Baets, posted 07 Mar 2021
The manuscript documents an interesting pilot study applying established machine learning approaches to classify ammonoids based on standard conch properties. I feel it is an important way forward to standardize and test ammonoid taxonomy, but some minor but crucial points need to be revised before I can officially recommend this manuscript.
The main points:
Focus on conch parameters: It is ok to focus on conch parameters as these are easier to get and analyze in a biologically meaningful way but a bit more discussion on why this is the case as well as how adding additional parameters (e.g., suture line, ornamentation such as ribbing) might improve statistical power to separate species would be crucial to discuss. Many species are not just defined by conch parameters so it would be crucial to point out that you are working with only a subset of characters used to define species which are more readily available in the literature and easier to analyze quantitatively (see also comments by reviewer 1).
Data: As pointed out in the manuscript, the dataset is limited to 11 species entered into the Paleobiology Database – the sample size of individual species are ok (> 50 – could still be better – some authors have suggested to have > 100 specimens available when including multiple ontogenetic stages, etc.). As an ammonoid worker which has worked on intraspecific variation – I can highlight that data for much more species would be available in the primary literature (a substantial part is still missing from the PDBD). I must admit that particularly in older literature measurements would need to be extracted from graphs and we still need to go some way before all paleontologists make this kind of data available as standard practice. Ideally, you should try to compile some additional data from the primary data to better understand what I mean and would help to broaden the scope of your analysis. As this is a pilot study, focusing on 11 species with samples > 50 could still ok, but it would be crucial to highlight which primary references yielded data for particular species. This also becomes crucial as for some species, data from multiple references are merged, presented data from multiple stratigraphic and geographic intervals (and likely also different degrees of preservation). This could for example explain the poorer performance for particular species like Owenites koeneni which derive from different localities and might also represent different preservations and ages. Please also, write species in italics as this is customary.
Performance of particular methods and species. The original authors might have assigned all their specimens to a particular species (e.g., Owenites koeneni) but mostly did not statistically evaluate how the conch parameters of their specimens compared with those of other localities and some even highlight qualitative differences with material from other localities. The homogeneity of conch parameters and there use to define species might therefore be to some degree compromised even before applying machine learning approaches. To place the performance of the methods into context for particular species, it would be crucial to add at least the primary reference providing data, their age range (single bed, biozone, etc.) as well the geographic scope (same locality, continent, etc.), so such potential issues could be glanced more transparently. In the discussion you focus on the performance of methods, but I would also be crucial to highlights which species are consistently picked up and which ones are not to better understand the impact of the issue of species definition. Which ones are often/sometimes merged and which ones are sometimes/often oversplit. This would allow a better discussion and understanding of how species definition and homogeneity of conch parameters might impact on the performance of the methods. At first glance, particularly Owenites koeneni seems to perform peculiarly and it is also one of the species which measurements deriving from several continents and publications. So it would be crucial to discuss this at greater length in the discussion
Code availability and reproducibility: It has been become standard practice to share the code at least upon publication (see Reviewer 2). Ideally, this should even be done during the review process as it would allow reviewers to verify the results, but I can to some degree understand the reluctance to do so before publication. Special repositories are however available for this purpose (GitHub) which allow to put embargos and restrictions on the availability of the data.
Please address these and other points raised by the reviewers and myself (see annotated pdf). I look forward to seeing the revised manuscript.
Download recommender's annotationsReviewed by anonymous reviewer 1, 05 Mar 2021
The manuscript provides a persuasive example of how to combine the morphological data of fossil taxa in existing databases with machine learning. Given how little machine learning is used in the paleontological sciences – despite the exponential growth of this computer science field – any contribution provides a valuable first step.
Overall, I have no major concerns with the work conducted. I am not familiar with all of the unsupervised methods used (listed in Table 5), though I have used the k-means approach in some of my previous papers. However, from my understanding of the literature, all the techniques applied are fairly standard in machine learning research, with many of these algorithms in use for over a decade.
My main concern with the manuscript is the reporting of the work. It has become routine in this field to publish code in a public repository like GitHub and to at least mention the platform used for the analysis (e.g. PyTorch) so that other can replicate, corroborate, and build on the work. The detail in this manuscript is very minimal. Detailed procedures would be especially helpful for the paleontological community, as it would give others guidance on how to apply machine learning to their own morphological datasets.
Additionally, while it appears that the results are promising, higher accuracies may be possible with new machine learning approaches, e.g. convolutional neural networks, and with a morphological dataset that perhaps includes more morphological features. Many of the morphological traits that we use in taxon identifications are chosen as much for their ease of measurement and reproducibility, as their diagnostic strength. Computer vision has the potential to expand the morphological traits we can use in taxonomic determinations.
In short, this is a solid first step in applying machine learning to fossil data. However, the methods used are not among the most cutting edge in a field that is evolving rapidly. I strongly urge the author to publish their code and for the PCI Paleo editors to make it part of the publication process.
Reviewed by Jérémie Bardin, 03 Feb 2021
This paper by Floe Foxon investigates the use of machine learning algorithms for ammonoid taxonomy. This is a really enjoying topic as ammonoid taxonomy suffers from many biases that could be avoided by using quantified methods to recognize taxa and identify species. The paper is clear and well-written. The “results” and “discussion” sections need reorganization and the discussion has to be expanded. I cannot properly evaluate the language as I am not an English native speaker. In my opinion, as the author mentions it, this study is more a proof-of-concept rather than a demonstration that machine learning may be useful with these parameters. Algorithms are well-parametrized and the procedures to quantify overfitting are ok.
Indeed, my main concern is that the somewhat good results in this paper come from the very low number of species treated. The author mentions as a limitation the low number of specimens for training but, to me, the real weakness is the number of species. Using machine learning methods for species identification of a higher number of species will require way more descriptors. The parameters used in this paper are the most common and the corresponding measurements are provided in the majority of papers describing ammonoids. Given that these measures are extremely available and that many people have important databases of such measures, I would have expected more than 11 species to demonstrate that these parameters with machine learnings methods could provide useful tools to identify ammonites and build a robust taxonomy.
Moreover, I would like to see more insights on the very general use of supervised vs unsupervised methods for ammonoids taxonomy. Supervised methods make the assumption that the target variable is true. These methods are thus suited to identify specimens given a robust taxonomy. Unsupervised methods, if performed on already settled taxonomy, quantify the congruence/difference between, on the one side, species definitions and taxonomic attributions of specimens and, on the other side, their morphological clustering. They should also be used to create taxonomy but there is much to do to properly include stratigraphical time, ontogeny and any kind of morphological features. All those points could be addressed in the discussion.
To conclude, I think this study afford the advantage of stimulating an underexplored topic even if the results are limited and the discussion would deserve a strengthening. Hereafter, some detailed comments.
Abstract - The verb “taxonomize” is rarley used. I am not sure of its meaning, I would recommend being more specific.
Introduction - Ammonoids is not the name of the subclass, it is Ammonoidea. Using Linnean nomenclature is very controversial now (even if still correct), I would replace “subclass” by “clade” or simply “group”. - The two parts of this sentence are somewhat redundant “ammonoids are crucial index fossils for biostratigraphy (Cox, 1995), therefore ammonoid taxonomy is useful for the study of stratigraphic subdivision”. - “conch morphology, coiling, and aperture shape.” Actually, coiling and aperture shape are parts of conch morphology. Better say: “conch morphology such as coiling, and aperture shape” - “ribs (their direction, spacing, and type) may be used for family classification”. I would remove “family”, ribs are useful at every level of taxonomy. By the way, I would also recommend removing Linnean ranks as much as possible. - Despite all the great things Dieter Korn did and does on ammonoids description, I am not sure he defined the numerous parameters in its 2010 paper as written. It seems to me that its contribution is more a summary and formalization. - “Since ammonoids exhibit intraspecific variation (De Baets et al., 2015), it follows that each species has a typical range of conch proportions which are diagnostic of taxonomy.” This is one of the main problems. Ammonite species are usually not built on advanced quantitative diagnoses. Most of the time, several species (usually a lot) will have overlapping morphologies. Moreover, many species are partly defined on stratigraphy itself. I think that the way ammonites’ species are built and the variability in this practice are of prime importance to properly use machine learnings algorithms. - Supervised (eg discriminant analyses) and unsupervised (clustering) methods have already been used on ammonoids, I expect the introduction and/or the discussion to review what has already been done on the topic (e.g. Hohenegger and Tatzreiter 1992, Meister et al. 2011, Bardin et al. 2015). - To me, the priority is to define robust species by the use of clustering methods given a clear definition of the paleontological species. For now, I think that using supervised algorithms on few parameters is largely flawed due to problems in current species definitions.
Data - The number of specimens and species may be a weakness of this work. I am not surprise to see such results for 11 species. - The author mentions in the “Limitations” section that he was not able to differentiate juveniles and adults but given the fact the diameter is used, there are ways to infer it.
Discussion - “A nearest neighbours algorithm was then implemented to calculate the average distance between each point and its m nearest neighbours”. Use m as defined before. Supervised models - A large part of the discussion would be better suited to the results section. Please reorganize the two sections (Results and Discussion). - I don’t understand the comparison of test accuracies to the accuracy of majority class prediction. Could you be more specific and explain why it is your baseline hypothesis? - A naïve question: is the test accuracy sufficient to choose between methods or do we need to consider the difference between test and train accuracies. In other words, do we care about an important over-fitting is the test accuracy is really good?
Additional references: - Bardin J, Rouget I, Benzaggagh M, Fürsich FT and Cecca F. 2015. Lower Toarcian (Jurassic) ammonites of the South Riffian ridges (Morocco): systematics and biostratigraphy. Journal of systematic paleontology. 13 (6). 471-501. - Hohenegger J and Tatzreiter F. 1992. Morphometric methods in determination of ammonite species, exemplified through Balatonites shells (Middle Triassic). Journal of Paleontology 66(5): 801-816. - Meister C, Dommergues JL, Dommergues C, Lachkar N and El Hariri K. 2011. Les ammonites du Pliensbachien du jebel Bou Rharraf(Haut Atlas oriental, Maroc). Geobios. 44. 117.e1–117.e60.